At VMware Explore EU 2024, the session “Unlocking Your Data with VMware by Broadcom and NVIDIA — RAG Deep Dive” delivered fascinating insights into the power of Retrieval Augmented Generation (RAG). Led by Frank Denneman and Shawn Kelly, this session explored how combining large language models (LLMs) with proprietary organizational data can revolutionize data utilization in enterprises.
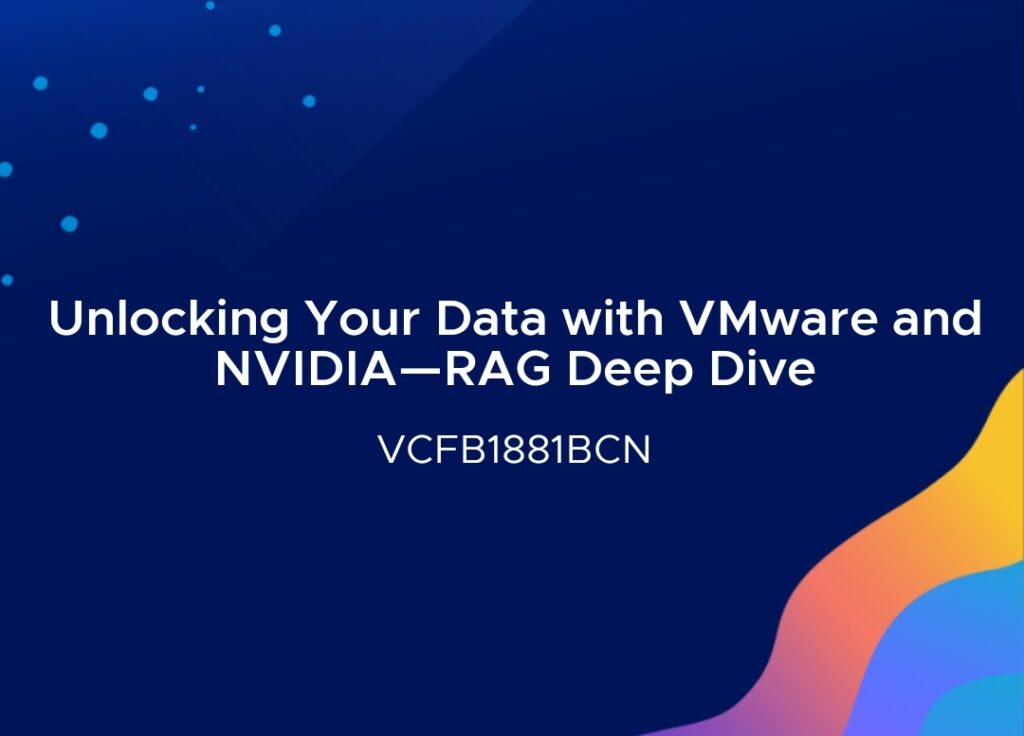
What is RAG?
RAG combines the strengths of LLMs with a Vector Database to enhance AI applications by integrating them with an organization’s proprietary data. This synergy allows for more precise and context-aware responses, crucial for business-critical operations.
Why RAG Matters:
- Enhanced Accuracy: Unlike traditional LLMs prone to “hallucinations” or inaccuracies, RAG provides validated, up-to-date answers by sourcing information directly from relevant databases.
- Contextual Relevance: It seamlessly blends general knowledge from LLMs with specific proprietary data, delivering highly relevant insights.
- Traceability and Transparency: RAG solutions can cite the documents used to generate responses, addressing one of the significant limitations of traditional LLMs.
How RAG Works:
- Data Indexing: Proprietary data is pre-processed and stored in a vector database.
- Question Processing: When a query is made, it is semantically embedded and matched against the vector database.
- Answer Generation: The most relevant data is retrieved and used to generate a precise answer.
Integration with NVIDIA:
NVIDIA’s Inference Microservice (NIM) accelerates this process by optimizing LLMs for rapid inference, leveraging GPU-accelerated infrastructure to enhance throughput and reduce latency.